Model-based optimization for efficient use of resources and energy.
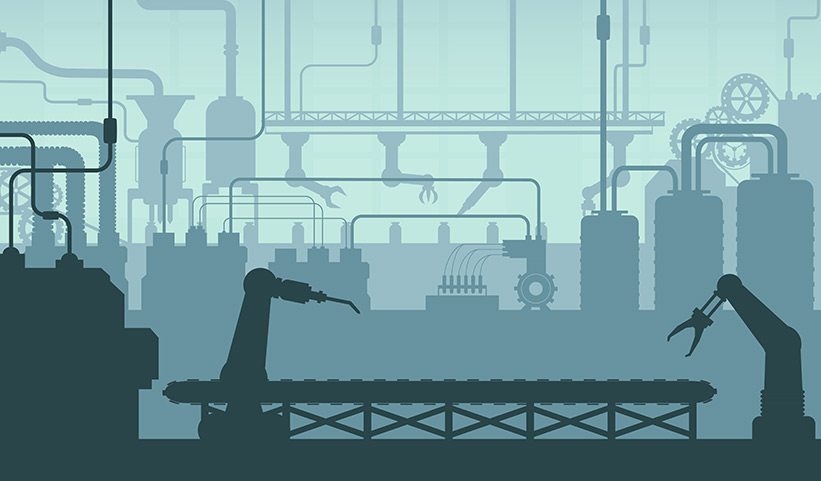
Project MORSE: Model-based optimization for efficient use of resources and energy.
The process industry is continuously looking for new ways to improve resource efficiency due to high dependence on resources (energy, raw materials, and utilities). In large scale production even small changes in using raw materials and in energy can significantly improve process efficiency.
The MORSE approach is to adopt new software tools for model-based predictive control, multi-criterial through process optimization and quality management with overall process coordination. The application of these new software tools leads to process improvements – reducing the use of raw material and energy while increasing the high quality and production rates.
The MORSE project aims to further develop and to integrate a set of software tools that have partly already been validated in different process steps in steel industries. These software prototype tools and models were developed and evaluated by six R&D partners of the consortium in collaboration with three process industry partners. With the enhanced MORSE tools companies of the process industry will be enabled to optimize the use of raw materials and energy by coordinated prediction and control of resource input and product quality along the entire process route from raw material and energy intake to customer delivery.
The mission of the MORSE project is to develop model-based, predictive raw material and energy optimization tools for the whole process route. This approach is demonstrated in steel industry, to increase yield and product quality in production of high-strength carbon steels, stainless steels and cast steels.
Our main tasks
- Use case definitions and targets
- System requirements
- Benchmark and performance indicators
- Layout of process models
- Initial release of process models
- Modifications of process models for use in plant-wide applications
- Validation of models based on historic plant and process data
- Self-learning capabilities for on-line application of process models
- Definition of processes and optimization targets
- Optimisation methods for through-process solutions
- Optimisation methods for unit process solutions
- Validation of optimisation methods
- Implement communication protocol between through-process layer and unit processes.
- Develop online monitoring and control for unit processes
- Iterative versions of through-process solutions implemented
- Iterative versions of unit process solutions implemented
- Evaluation of results (carbon steel, stainless steel and cast steel use cases)
Partners
BFI | CYBERNETICA | GRIPS | IDENER.AI | MFL | OUTOKUMPU | SSAB | SWD | VTT
Start date – finish date
10 / 2017 - 02 / 2022
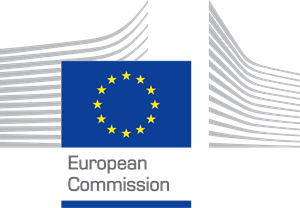
This project has received funding from the European Union’s Horizon 2020 research and innovation programme under grant agreement Nº 768652